In commerce, companies often consider cutting marketing budgets during economic uncertainty or cost-cutting efforts. However, this short-term approach can harm long-term growth and market competitiveness.
Consistent marketing is vital for maintaining visibility and brand awareness, which are costly to rebuild once lost. It also fosters trust and credibility by showcasing the company’s expertise and values.
To maximize the efficiency of fixed marketing budgets, attribution models help businesses evaluate the impact of various touchpoints in the customer journey. These models guide optimal budget allocation by identifying the most effective channels for driving conversions and revenue.
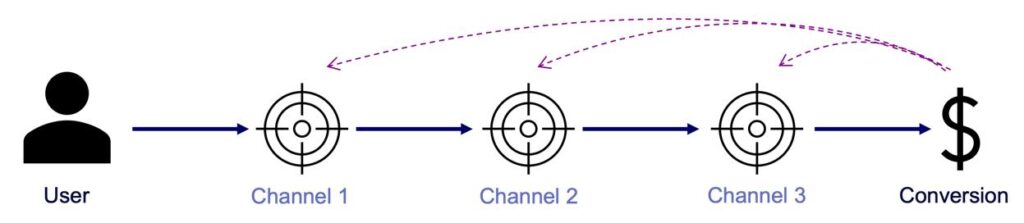
Companies use a mix of marketing strategies like email, SEA, SEO, affiliate marketing, and social media. Attribution models analyze customer interactions across these channels, providing key insights into the customer journey.
Key Benefits of Attribution Models:
- Customer Insights: Identify the most effective channels to boost engagement and conversions.
- Optimized Budgets: Allocate resources to high-ROI channels for maximum impact.
- Data-Driven Decisions: Enable informed strategies that align marketing and sales efforts.
In online advertising, evaluating media effectiveness is essential as costs depend on clicks or impressions. Multi-channel attribution models help assess each channel’s impact, ensuring efficient budget use, improved ROI, and successful marketing outcomes.
Read the full article by Dr. Alexander Lammers to get to know all kinds of taditional and dynamic attribution models common in commerce: https://data-science-blog.com/blog/2024/10/20/data-driven-attribution-modeling/
Markov Chain based Attribution Modelling
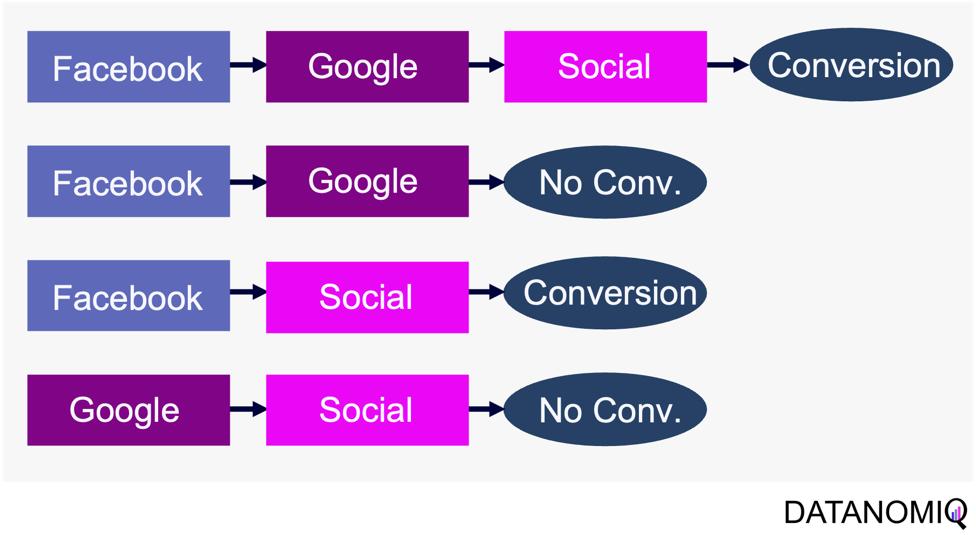
The model calculates the conversion likelihood by examining transitions between touchpoints. Those transitions are depicted in the following probability tree.
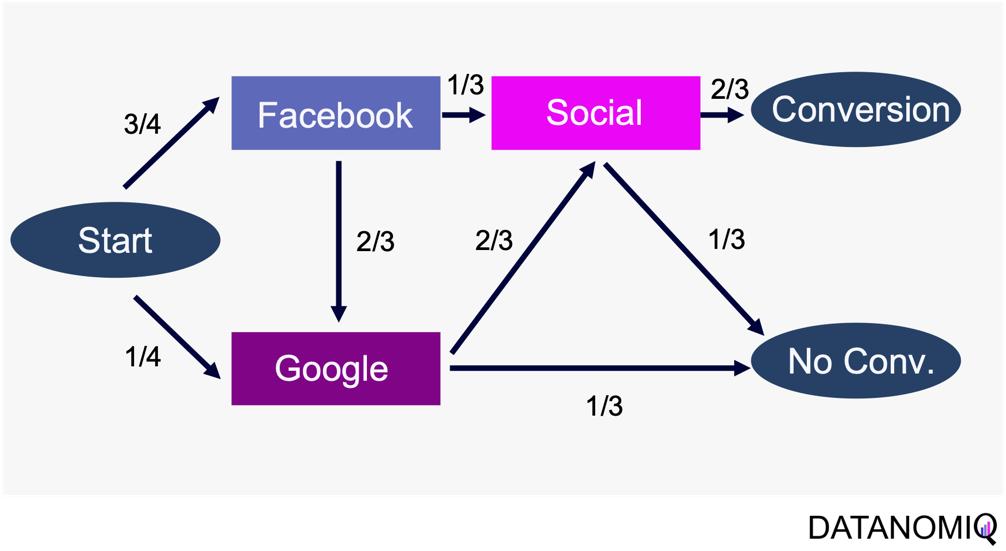
Using the network structure, a transition matrix can be constructed to quantify the influence of each touchpoint, highlighting the significance of each channel.
Markov chain attribution accounts for the sequential nature of customer journeys and uses historical data to estimate transition probabilities, reflecting real customer behavior. It allows flexibility by incorporating factors like time decay, channel interactions, and customizable attribution rules.
This method can also extend to higher-order chains, where transition probabilities depend on multiple prior states, enabling a deeper analysis of customer behavior. By introducing a memory parameter (assumed to be zero here), Markov chain attribution provides a robust framework for evaluating the impact of marketing touchpoints.
Deep Learning for Attribution Modelling
Customer journey data often suffices for evaluating channel contributions but can be enhanced with additional analytics data from various sources. Companies may leverage data such as website activity (clicks, page views, conversions), UTM parameters (source, medium, campaign), device type, location, user engagement metrics, and scroll frequency.
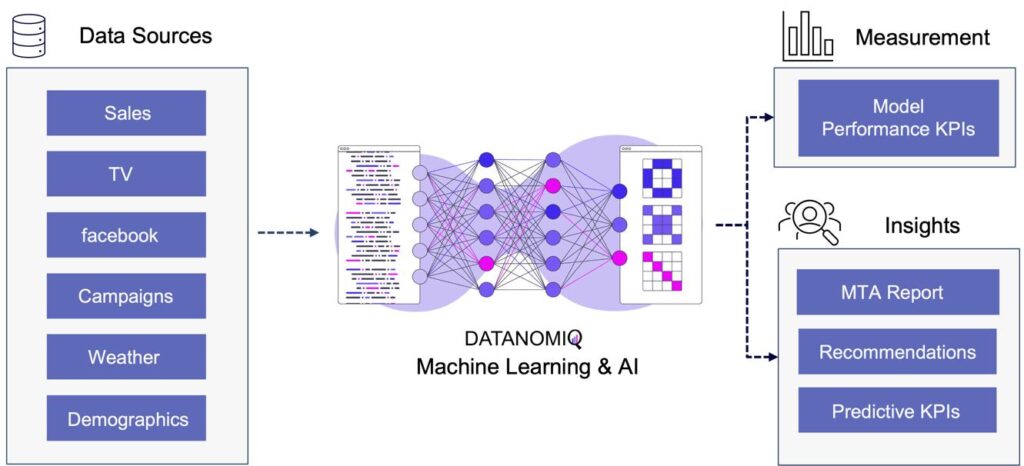
This data can train binary classification models to predict conversion probabilities at each step in a multi-touch attribution (MTA) model, identifying effective and overvalued channels. Common methods include logistic regression, gradient boosting for unbalanced data, random forests, and SVMs. For complex, sequential data, deep learning models like LSTMs and Transformers are used to capture long-range dependencies among touchpoints.
The approach is scalable, capable of handling large volumes of data, making it ideal for organizations with extensive marketing campaigns and complex customer journeys. By leveraging advanced algorithms, it offers more accurate attribution of credit to different touchpoints, enabling marketers to make informed, data-driven decisions.
All those models are part of the Machine Learning & AI Toolkit for assessing MTA. And since the business world is evolving quickly, newer methods such as double Machine Learning or causal forest models that are discussed in the marketing literature (e.g. Langen & Huber 2023) in combination with eXplainable Artificial Intelligence (XAI) can also be applied as well in the DATANOMIQ Machine Learning and AI framework.
Business Logic should always be considered!
The true value of attribution modeling lies not just in applying the best theoretical concepts—discussed below—but in aligning them with the firm’s business logic. Effective modeling ensures companies optimize budget allocation while focusing on a long-term growth strategy that aligns with their goals.
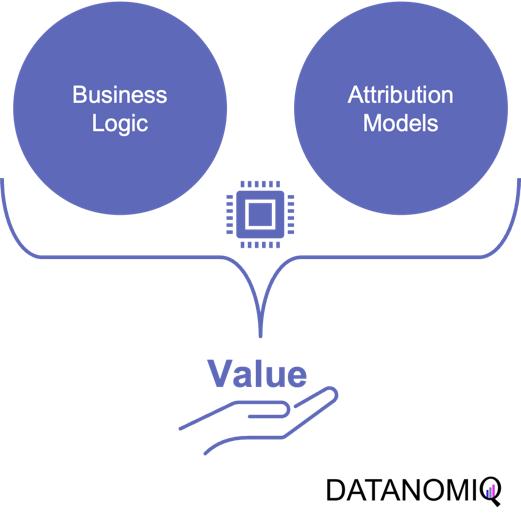
Incorporating business logic into attribution models is a critical yet often overlooked step. It is essential for unlocking the full potential of attribution modeling and enabling data-driven decisions that align with business objectives. Without this integration, even the most advanced models risk producing insights that fail to drive meaningful or effective marketing strategies.
DATANOMIQ is the independent consulting and service partner for business intelligence, process mining and data science. We are opening up the diverse possibilities offered by big data and artificial intelligence in all areas of the value chain. We rely on the best minds and the most comprehensive method and technology portfolio for the use of data for business optimization.